Botnet attack analysis of Deflect protected website bdsmovement.net
This report covers attacks between February 1st and March 31st of six discovered incidents targeting the bdsmovement.net website, including methods of attack, identified botnets and their characteristics. It provides detailed technical information and analysis of trends with the introduction of the Bothound library for attack fingerprinting and botnet classification. We cluster malicious behaviour on the Deflect network to identify individual botnets and employ intersection analysis of their activity throughout the documented incidents and further afield. Our research includes discovered patterns in the selection of targets by the actors controlling these attacks.
Deflect is a website security project working with independent media, human rights organizations and activists. It offers DDoS mitigation, secure hosting and attack analytics, free of charge to qualifying organizations. All of our tools are open source and we operate according to principles promoting the privacy of our clients. Deflect is a project of eQualit.ie, a Canadian not-for-profit organization working to promote and defend human rights in the digital age.
Navigation links: Attack Profile; Botnet profile; Botnet target selection; Botnet behaviour comparison; In-depth incident analysis; Report conclusions
General Info
The Boycott, Divestment and Sanctions Movement (BDS Movement, bdsmovement.net) is a Palestinian global campaign, initiated in 2005. The BDS movement aims to nonviolently pressure Israel to comply with international law and to end international complicity with Israel’s violations of international law. Their website has been protected by Deflect since late 2014 and has frequently been attacked.
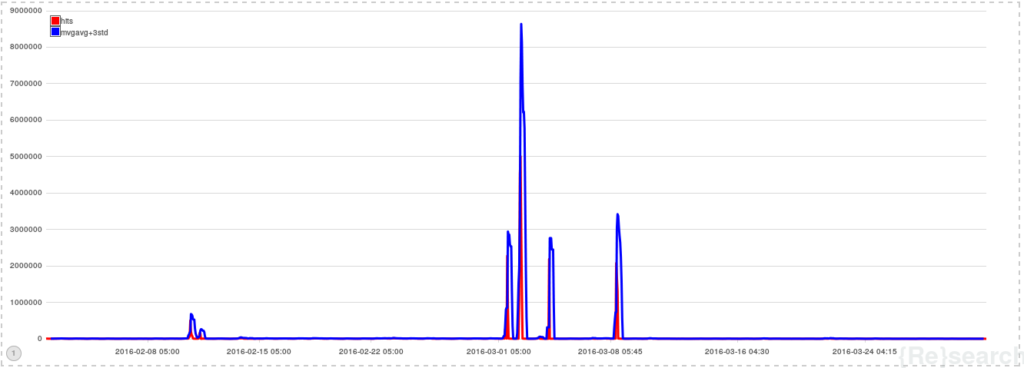
Graph 1. Timelion graph showing the average hits per day in the period of February 1st to March 31st (in red) and the moving average + 3 standard deviation (in blue).
Attack Profile
During February and March of 2016, there were 6 recorded incidents against the target website. The Deflect Labs infrastructure allows us to capture, process and profile each attack, analysing unique incidents and intersecting findings with a database of profiled botnets. We define the parameters for anomalous behaviour on the network and then group (“cluster”) malicious IPs into botnets using unsupervised machine learning algorithms.
We define each incident by wrapping it inside a given time frame, record the total number of hits that reached the website during this time and use our analytic tool set to separate malicious requests made by bots from genuine everyday traffic.
Table 1. Attacks Summary, including start/end date, duration, size of the incident, size and number of the botnets detected
id | Incident Start | Incident Stop | Duration | Total hits | Unique IPs | No. of bots identified | Identified botnets |
29 | 2016-02-10 21:00 | 2016-02-11 01:00 | ~5hrs | 879,634 | 14,773 | 12,921 | 3 |
30 | 2016-02-11 10:30 | 2016-02-11 12:30 | ~2hrs | 321,203 | 11,108 | 9,023 | 3 |
31 | 2016-03-01 15:00 | 2016-03-01 19:30 | ~6h30 | 3,597,689 | 5,918 | 3,243 | 3 |
32 | 2016-03-02 12:30 | 2016-03-02 16:00 | ~3h30 | 13,559,169 | 19,851 | 2,748 | 2 |
33 | 2016-03-04 09:00 | 2016-03-04 09:30 | ~30min | 2,058,710 | 9,613 | 8,844 | 1 |
34 | 2016-03-08 14:20 | 2016-03-08 16:40 | ~2h20 | 5,017,045 | 7,937 | 7,151 | 1 |
The number of unique bots and their grouping into specific botnets is the result of clustering work by BotHound. This toolkit classifies IPs by their behaviour, and allows us to determine the presence of different botnets in the same incident (attack).
Botnet profile
Using BotHound, we have calculated the percentage of unique IPs (classified as bots) that recur in separate incidents. A substantial percentage of previously seen bots would be one way to identify whether a botnet was re-used for attacking the same target. It would reveal a trend in botnet command and control behaviour. This intersection of botnet IPs also creates an opportunity to compare activity between several target websites, whether protected by Deflect or on one of our peers’ networks. Taken together, we begin to build a profile of activity for each botnet, helping us make assumptions about their motivation and target list.
Incident # |
No. of identical bots |
The portion of identical bots |
29, 30 | 6,928 | 76.8% |
31, 32 | 1,450 | 91.0% |
33, 34 | 4,249 | 59.4% |
32, 33 | 438 | 17.9% |
Table 3. Identified botnets and the incidents they appear in
Botnet ID | Seen in incident | Unique bots | Top 10 countries of bot origin | Attack method |
1 | 29, 30 | 13,857 | Russian Federation; Ukraine; China; Lithuania; Germany; Switzerland; Gibraltar; United Kingdom; Netherlands; France | POST |
2 | 29, 30 | 8,913 | Russian Federation; China; Ukraine; Germany; Lithuania; United States; Switzerland; United Kingdom; France; Gibraltar | POST |
4 | 31, 32 | 2,589 | United States; Germany; United Kingdom; Netherlands; China; Japan; Singapore; Ireland; France; Spain; Australia | Pingback |
5 | 31, 32 | 772 | United States; United Kingdom; Germany; Netherlands; Italy; France; Russian Federation; Singapore; Canada; Japan; China | Pingback |
6 | 31 | 971 | United States; China; Germany; Japan; United Kingdom; Singapore; Netherlands; France; Ireland; Canada; Australia | Pingback |
7 | 33, 34 | 11,746 | United States; United Kingdom; Germany; France; Netherlands; China; Canada; Russian Federation; Ireland; Spain; Turkey | Pingback |
Botnet target selection
Deflect protects a large number of qualifying human rights and independent media websites the world over. Our botnet capture and analytic tool set allows us to investigate attack characteristics and patterns. We consider the presence (intersection) of over 30% of identical bots as originating from a similar botnet. During our broader analysis of the time period covered by this report, we have found that botnet #7, which targeted the bdsmovement.net website on March 3rd, also hit the website of an Israeli Human Rights organisation under our protection on April 5th and April 11th. In each incident, over 50% of the botnet IPs hitting this website were also part of botnet #7 analysed in this report. Furthermore, a peer website security organization reviewed our findings and concluded that a substantial amount of IPs belonging to this botnet were targeting another Israeli media website under its protection, on April 7th and April 12th. Organisations targeted by this botnet do not share a common editorial or are in any way associated with each other. Their primary similarities can be found in their emphasis on issues relevant to the protection of human rights in the Occupied Territories and exposing violations in the ongoing conflict. Our analysis shows that these websites may have a common adversary — the controller or renter of botnet #7 — that their individual work has aggrieved. We will present our findings on this investigation in more detail in an upcoming report.
Botnet behaviour comparison
BotHound works by classifying the behaviour of actors on the network (whether human or bot) and clustering them according to a set of pre-defined features. Malicious behaviour stands out from the everyday trend of regular traffic. On the picture below the RED spots refer to attacker sessions, while BLUE spots refer to all other (regular traffic). The graphic displays all the 6 incidents combined. We chose the following 3 dimensions to visually represent a projection from a 7-dimensional space (where BotHound clustering is calculated):
- HTTP request depth
- Variance of HTTP request interval
- HTML to image ratio
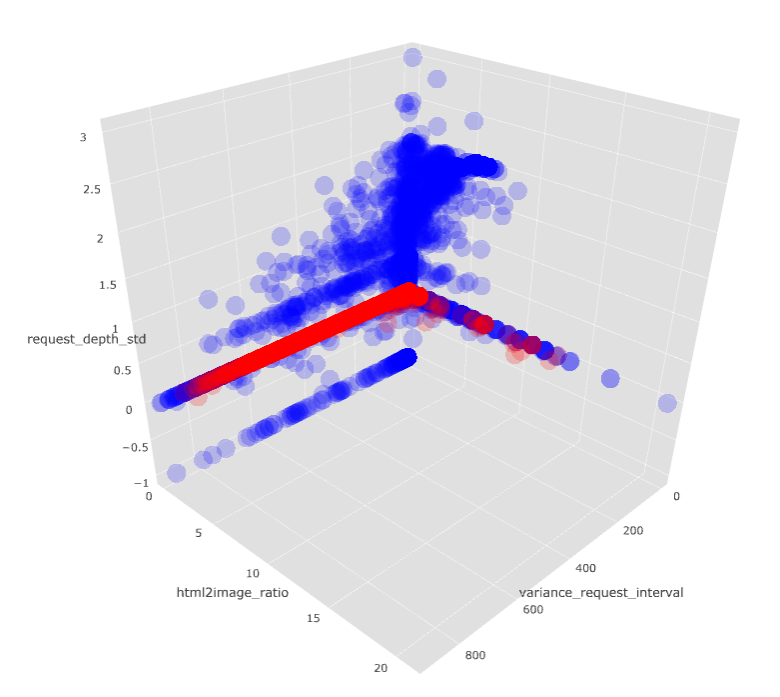
Graph 5. Clustering of bot behaviour from the six incidents covered in this report. The graphic illustrates that malicious behaviour, no matter the botnet characteristics, follows a determined pattern which resembles automated machine-driven properties of a botnet attack.
In-depth incident analysis
We have captured, analysed and now profiled each botnet witnessed in the 6 incidents. We break down incidents into three groups, by similarity of attack characteristics and the time of occurrence.
Incidents #29 & #30
Date: February 10-11, 2016
Duration: approximately 28 hours
Identified botnets: 2 (botnet id: #1 #2)
IP intersection between botnets: 76%
Attack type: HTTP POST
Attack analysis
After doing extensive cluster analysis to separate “good” from “bad” IPs based on their behaviour during the incident time frame, we applied a novel secondary clustering method which identified two different patterns of behaviour spanning both incidents. The first attack pattern was using bots to hit the target very fast, with similar characteristics (session length, request intervals, etc.). The second botnet was hitting slower, but more consistently. The session length was varying, likely to evade our mitigation mechanisms. However, the request interval between hits was zero, which helped us identify them. It is easy to distinguish two different botnets from the graphs below.
Identified botnet #1
Members: 13,857
Observations:
- Session length = 314 sec
- Payload average = 521 byte
- Hit rate = 0.04 /minute
- Requests: 500,000
- Host header: accurate
- Method: POST (> 99.9%)
- URI path: / (> 99.9%)
- UA: low variation, with most major UAs represented
Deflect Response: Moderate blocking success, origin was affected.
Members: 8,913
Observations:
- Session length = 429 sec
- Payload average = 447 byte
- Hit rate = 0.05 /minute
- Requests: 600,000
- Host header: accurate
- Method: POST (> 99.9%)
- URI path: / (> 99.9%)
- UA: low variation (slightly higher than botnet 1), most major UAs represented
Deflect Response: Moderate blocking success, origin was affected.
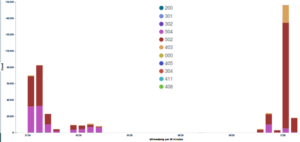
The botnet utilises several hundred unique IPs and a few dozen rotating user agents
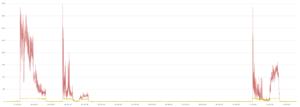
The botnet attacks with several hundred unique IPs and rotates through a few dozen user agents. Graph tallies at 15 second intervals.
IP geo-reference
The IP address requesting a site can be geo-located. Another way we visualize botnet behavior is by cross-referencing the country of bot origin. We can easily see attack intensity (number of hits) versus bot distribution (unique IPs) in the diagrams below.
User agent and device
Every website request usually contains a header with identifying information about the requester. This can be faked, of course, but in any case stands out from the general pattern of traffic to the website. These incidents had a high consistency of “Generic Smartphone” and “Other” devices – describing the hardware unit from which the request was supposedly made. It is common for botnets to spoof a user agent device or, at least, share a common one.
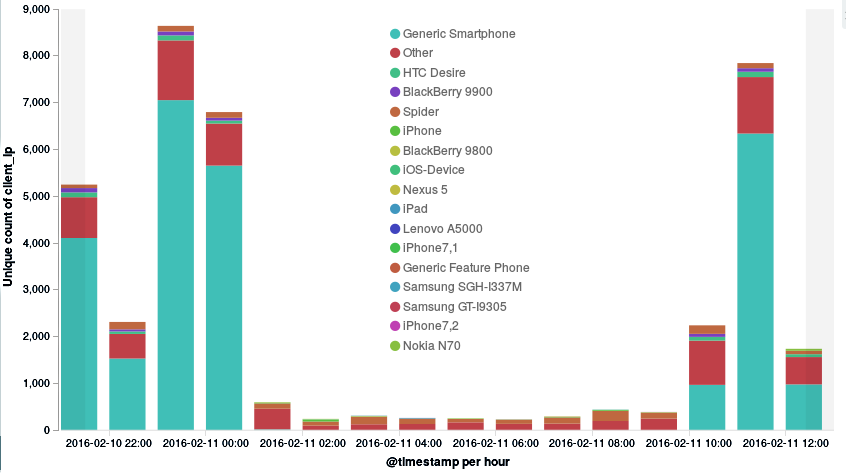
Graph 8. Shows the devices used in the February botnet attack. As we can see, the majority comes from “Generic Smartphone” or “Other” device. Such consistency shows that these are part of an attack, rather than regular visitors.
Conclusions on incident #29 and #30 attacks
- These attacks were distinguished by the relatively large number of participating bots, but were smaller in intensity (number of hits on target) compared to incidents #31-34. Three attacks were launched during the period of these incidents, requesting the same url ( /- ), as well as using the same “device” in the user agent of the request.
- There were two and possibly three botnets in these incidents. They can be differentiated by the geographic location of their bots and hit rates during attack. What is interesting is that the attack method between the different botnets and attack times is the same. Also the two botnets share a high percentage of intersecting bot IPs (76.8%). This may be an indication that they are subnets of a larger malicious network and are being controlled by the same entity.
Incidents #31 & #32
Date: March 1-2, 2016
Duration: approximately 21.5 hours
Identified botnets: 3 (botnet id: #4 #5 #6 )
IP intersection between botnets: 91%
Attack type: Reflection – WordPress Pingback[1]
Attack Analysis
Attackers utilised the same botnet (91% intersection) during incidents #31 and #32 within a time range of 22 hours. Incident #32 is the biggest in terms of hits out of the entire period covered by this report – counting over 13.5 million total hits in 6 hours. These incidents have a very similar UA (device) characteristic, the majority of which are identified as “Spider” (we are making an intersectional analysis on the UA further down in this report).
Members: 2,589
Observations:
- Session length = 2,971 sec
- Payload average = 8,217 byte
- Hit rate = 1.7 /minute
- Requests: 10.8 million
- Host header: accurate
- Method: GET (> 99%)
- URI path: / (> 99%)
- UA: high variation, all WordPress pingback
Deflect Response: Successfully blocked. 91% of responses to botnet processed by edge within 20ms
Members: 772
Observations:
- Session length = 3,587 sec
- Payload average = 10,221 byte
- Hit rate = 0.48 /minute
- Requests: 3 million
- Host header: accurate
- Method: GET (> 99%)
- URI path: / (> 99%)
- UA: high variation, all WordPress pingback
Deflect Response: Successfully blocked. 85% of responses to botnet processed by edge within 20ms
Members: 971
Observations:
- Session length = 583 sec
- Payload average = 31,317 byte
- Hit rate = 0.49 /minute
- Requests: 145,000
- Host header: accurate
- Method: GET (> 99%)
- URI path: / (> 99%)
- UA variation: high variation, all WordPress pingback
Deflect Response: Relatively small incident – some attackers did not trigger our early detection with around 15% getting through to origin (22,000 requests returned an HTTP 200). Successfully blocked.
User agent and device
The “UA” parameter in our logging system identifies the user agent string in the request header made to the target website. It usually represents the signature (or version) of the program used to query the website, for example “Mozilla/5.0 (Windows NT 6.1; WOW64; Trident/7.0; rv:11.0) like Gecko” means that the request was made from Internet Explorer version 11, running on the Windows 7 operating system [2]. The “device” parameter in our logging system identifies the hardware (device) the user agent is running on, for example “iOS Device” or “Nexus 5” or “Windows 7”. In this case, the vast majority of IP addresses hitting the site were categorised as “spiders”. A spider, or web crawler, is software used by search engines to index the web. User agent strings are just text and can be changed (faked) to say anything – including copying a user agent string commonly used by some other software.
Conclusions on incident #31 and #32 attacks
- These incidents stand out for their common attack and attacker characteristics, with an intersection of 91% of bots used in both instances (of the smaller incident). Botnet #4 and #5 behaviour differs only in their hit rate. Botnet #5 and #6 have a similar number of bots and an almost identical hit rate. Interestingly, they differ greatly in the number of hits each one of them launched at the target site. It seems that all three botnets had strong presence on computers in the United States. All botnets used the same attack method – WordPress pingback – in both incidents.
- The similarities between bot IP addresses and the attempts to vary the attack pattern from very similar botnets indicates human lead efforts to adapt their botnet to get past Deflect defences. It appears that the botnets used in these two incidents have the same controller behind them.
Incidents #33 & #34
Date: March 4, March 8, 2016
Duration: 30 mins, 2 hours and 20 minutes
Number of bots: 8,844 and 7,151
Identified botnets: 1 (botnet id: #7)
Attack type: Reflection – WordPress Pingback[1]
Identified botnet #7
Members: 11,746
Observations:
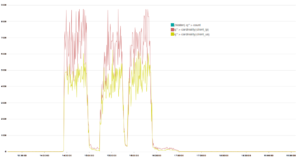
Graph 11. Comparable values of unique IPs and unique UAs. We see a huge difference from other botnets in this report
- Session length = 2,665 sec
- Payload average = 15,572 byte
- Hit rate = 0.30 /minute
- Requests: 7.9 million
- Host header: accurate
- Method: GET (> 99%)
- URI path: / (> 99%)
- UA variation: high variation, mostly WordPress pingback (92%)
Deflect Response: Moderate blocking success. 75% of requests dealt with in <200ms, 5% origin read timeouts
Conclusions on incident #33 and #34 attacks
- Incident #33 comes across as a probe (or a first attempt) before a much stronger attack with similar characteristics is launched in incident #34. This is backed up by the use of a single botnet in both incidents.
- Botnet #7 appears in other attacks against Israeli websites, on our network and on the network of one of our peers. The attack pattern used in these incidents is similar to the previous two incidents, and we have found a 17.9% intersection between bots used in incidents #32 and #33, possibly linking #31-34 together. Along with the prevalence of bots originating from the United States, there is some justification that botnets 4-7 originate from a similar larger network.
Report conclusions
Attempts to bring down the bdsmovement.net website were made using several (at least two distinct and relatively large) botnets and varied in their technical approach. This shows a level of sophistication and commitment not generally seen on the Deflect network. The choice of attack method allowed us to see which website was being targeted, which may have been a conscious decision. However, we did not find anything linking attacks in incidents #29-30 with attacks in incidents #31-34. Relative success with affecting the origin in the first two incidents was not built upon in the next four. Furthermore, other effective methods to swarm the network with traffic or overwhelm our defence mechanisms could have been used, had the attackers had enough resources and dedication to achieve their aims.
The creation of historical profiles for botnet activity and the ability to intersect our results with peer organizations will lead to better understanding of trends, across a greater swath of the Internet. Adapting botnet classification tooling to automated defense mechanisms will allow us to notify peers about established and confirmed botnets in advance of an attack. By slowly chipping away at the impunity of botnet controllers, we hope to reduce the prevalence of DDoS attacks as a method for suppressing online voices.
eQualit.ie is inviting organizations interested in this collaboration to reach out.
[1] A WordPress pingback attack uses a legitimate function within WordPress, notifying other websites that you are linking to them, in the hope for reciprocity. It calls the XML-RPC function to send a pingback request. The attacker chooses a range of WordPress sites and sends them a pingback request, spoofing the origin as the target website. This feature is enabled by default on WordPress installations and many people run their websites unaware of the fact that their server is being used to reflect a DDoS attack.
[2] http://www.useragentstring.com/index.php